- Home >
- Publications >
- Domain-invariant features for mechanism of action prediction in a multi-cell-line drug screen
Domain-invariant features for mechanism of action prediction in a multi-cell-line drug screen
Authors
Joseph C Boyd, Alice Pinheiro, Elaine Del Nery, Fabien Reyal, Thomas Walter
Abstract
Abstract
Motivation
High-content screening is an important tool in drug discovery and characterization. Often, high-content drug screens are performed on one single-cell line. Yet, a single-cell line cannot be thought of as a perfect disease model. Many diseases feature an important molecular heterogeneity. Consequently, a drug may be effective against one molecular subtype of a disease, but less so against another. To characterize drugs with respect to their effect not only on one cell line but on a panel of cell lines is therefore a promising strategy to streamline the drug discovery process.
Results
The contribution of this article is 2-fold. First, we investigate whether we can predict drug mechanism of action (MOA) at the molecular level without optimization of the MOA classes to the screen specificities. To this end, we benchmark a set of algorithms within a conventional pipeline, and evaluate their MOA prediction performance according to a statistically rigorous framework. Second, we extend this conventional pipeline to the simultaneous analysis of multiple cell lines, each manifesting potentially different morphological baselines. For this, we propose multi-task autoencoders, including a domain-adaptive model used to construct domain-invariant feature representations across cell lines. We apply these methods to a pilot screen of two triple negative breast cancer cell lines as models for two different molecular subtypes of the disease.
Availability and implementation
https://github.com/jcboyd/multi-cell-line or https://zenodo.org/record/2677923.
Supplementary information
Supplementary data are available at Bioinformatics online.
Members
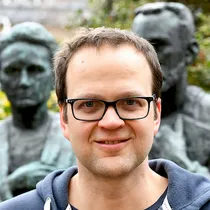