Team
Computational Systems Biology of Cancer
Presentation
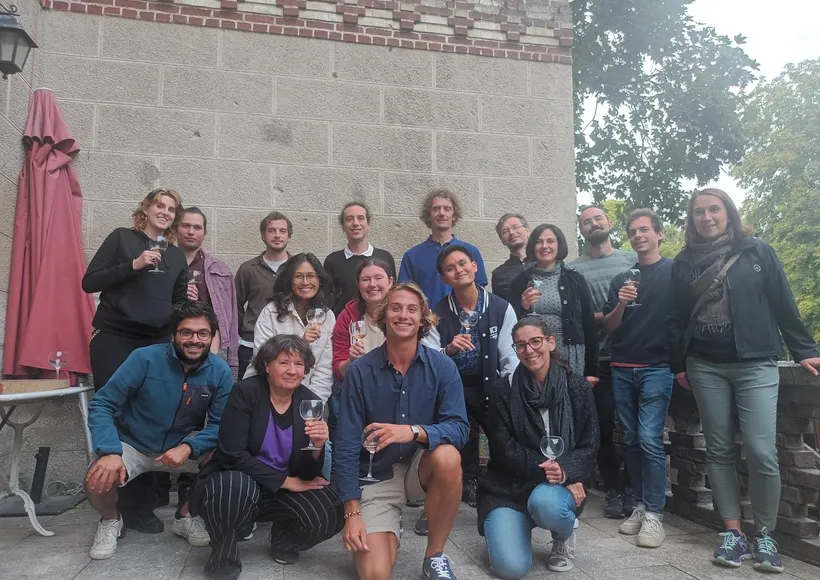
Our research aims at deciphering the molecular determinants of cancer and make this knowledge available to improve patient management. It is based on high-dimensional and multi-level omics tumor profiles, and proceed by sophisticated machine learning approaches as well as biological network modelling.Â
As a part of our research, we are developing software which is publicly available on our team GitHub repository : https://github.com/sysbio-curie.
At Institut Curie our situation is ideal to pursue these goals, since the choice of collaborations with biologists and clinicians, and the many technological core facilities of the institute offer many options to set up original and cutting-edge projects. We are also involved in many national and international (mainly European) projects with other laboratories in Spain, Germany, Italy, Norway, Netherlands, USA or Japan.
Four decades of cancer molecular biology research have led to the identification of many molecular determinants of this pathology, and showed that they are organized in pathways which in turn are tightly interconnected in a complex network of interactions which covers essential cell processes (proliferation, death, differentiation, DNA repair, immune response…). The existence of crosstalks, feedbacks and compensatory mechanisms invalidates simplistic reasoning and requires mathematical modeling to decipher how cell integrates signals to give rise to particular phenotypes, and to exploit this knowledge for rationalizing clinical treatments.
Another very important aspect of today’s biological and clinical cancer research lies in the high-throughput characterisation of tumours and their micro-environment, at the genome, transcriptome, proteome and epigenome levels (using technologies such as next-generation sequencing (NGS), single cell profiling, mass spectrometry, spatial transcriptomics…), as well as phenotypic level (using high-throughput imaging techniques). These large-scale technologies generate huge volumes of data, making cancer research a big data science.
The multi-dimensional nature of these data typically reaches tens of thousands if not millions of dimensions. This situation requires powerful machine learning approaches to disentangle the hidden signals and convert data into scientific knowledge. Another feature of our data if their multi-level essence, covering molecular, cellular, tissular and patient levels, and their diachronic nature, either through at molecular level or at tumor progression scale.
The volumes, completeness and resolution of the data makes it possible today to integrate them with our knowledge of the signalling circuitry of the cell to build personalized models of tumors which shed light on the functioning of the tumoral cell, how its fate is determined, and how to counteract deleterious phenotypes and propose personalized treatment, the so-called precision medicine approach. These goals have led us to propose new concepts and strategies falling within the field of computational systems biology of cancer.
The first step in the exploitation of high throughput molecular data consists in the extraction of a biological signal from measurements with a low experimental signal/noise ratio and conducted in parallel on thousands or millions of variables (genes, positions on the genome, etc.). This assumes that experimental artefacts be erased (data normalisation) and error rate controlled. We develop tools that achieve this goal in particular for NGS, taking into account the specificities of cancer when needed. Then the biological data must be converted into biological knowledge and e.g. any biological pathways involved in the disease identified. For this purpose, we also develop complexity reduction methods enabling the analysis of multi-dimensional data. These methods are based on mathematical theories like independent component analysis, elastic principal graphs, neural networks of many types, optimal transport, to name a few.
Understanding tumorigenesis and improving clinical strategies requires the detailed knowledge of the molecular interaction networks that control mechanisms of cell proliferation, death and differentiation. We construct an atlas of cell signaling of cancer (acsn.curie.fr), containing detailed map for cell cycle, DNA repair, EMT, programmed cell death and survival, immune response... We also develop computational tools for the analysis of networks, for example for integrating mutational and expression profiles of tumors, or for finding optimal intervention points for a particular tumor in a therapeutic perspective.
We then study the tumoral systems in a dynamic manner, building mathematical models of their molecular networks and proposing prediction of the effect of perturbations like mutations or drug compound. Through this approach, we are able to demonstrate essential system properties (typically global phenotype). Recent applications include finding new therapeutic target candidates in triple negative breast cancer, identifying synthetic interactions in DNA repair machinery, deciphering the modes of action of microRNAs, predicting cell fate decision to die or survive upon cell death receptor engagement, understanding the mechanisms of tumor invasion, proposing strategies for building relevant mouse models of colon cancer metastasis, or exploring the synergistic effects of immune checkpoint inhibitors.
Our mathematical models are then challenged with experimental data and iterative loops between modelling and experimentation allows model improvement and validation. In term of methodology, we use and develop innovative approaches based on literature analysis, complexity reduction, logical modelling, differential equation systems, robustness study, and multidimensional statistical analysis. We have developed a strong expertise in the abovementioned techniques, and put it in practice in many collaborative projects with biologists and clinicians, with a main focus on solid tumors (breast, bladder, uveal melanoma, colon, pediatric tumors…).